From providing liquidity to creating a near-sentient online companion, AI agents are taking on a broader range of tasks and functionalities in various activities, leveraging blockchain for storing data, decentralisation and interoperability, validating performance and creating a virtual economy within AI-agent platforms.
With so many developments nowadays, what are the top projects to watch, is it possible to invest in them, and how do they fit into the emerging landscape of AI agents? We answer these and related questions in this article to give you a wider perspective on what AI agents are out there, and what their applications are in the crypto market.
What are AI Agents?
While often mistaken for bots, AI agents are more advanced autonomous software that can perform complex tasks, make decisions, and learn over time with minimal human intervention. Unlike simple bots that merely suggest actions or provide information based on a predefined algorithm, AI agents act independently, executing tasks directly on a user’s behalf and evolving based on the data they process.
The short comparison between AI agents and bots is given below:
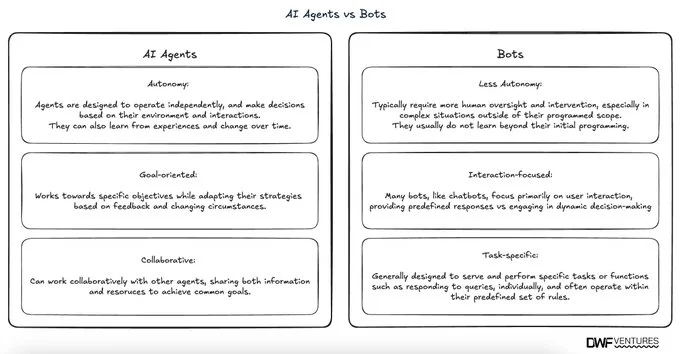
AI Agents | Bots |
---|---|
More autonomy: Agents are designed to operate independently and make decisions based on their environment and interactions. They can also learn from experiences and evolve over time. | Less autonomy: Bots typically require more human oversight and intervention, especially in complex situations outside of their programmed scope. They usually do not learn beyond their initial programming. |
Goal-oriented: Works towards specific objectives while adapting their strategies based on feedback and changing circumstances. | Interaction-focused: Many bots, like chatbots, focus primarily on user interaction, providing predefined responses without engaging in dynamic decision-making. |
Collaborative: AI agents can work collaboratively with other agents, sharing both information and resources to achieve common goals. | Task-specific: Generally designed to serve and perform specific tasks or functions such as responding to queries, individually, and often operate within their predefined set of rules. |
Types of AI Agents
AI agents are arising across various sectors, each designed for specific applications. We distinguish the four most popular AI agent types:
- Infrastructure.
- Agent Connectivity.
- DeFi.
- Interactivity.
Each category serves a distinct purpose, enhancing the functionality and potential of AI agents within its domain. You can see the most significant projects for all AI agent types in the table below:
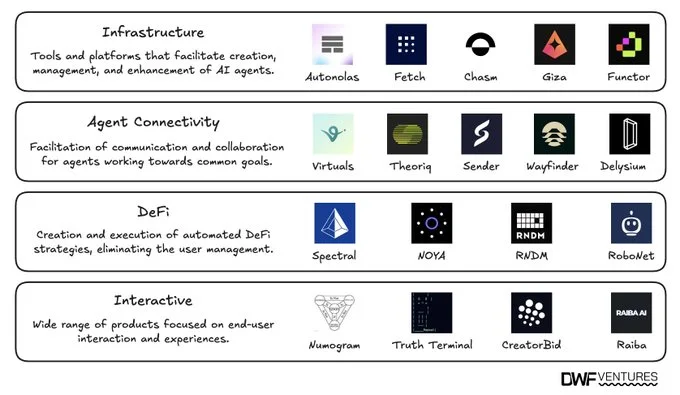
Infrastructure: Autonolas
The first in our list is Autonolas (OLAS) Network, a web3 project that provides a robust infrastructure of interchangeable software components. These components are building blocks that developers can use to create custom AI agents, tailoring them to specific tasks or requirements.
Each module serves a certain function like data processing, decision-making, or communication, and can be added, removed, or modified to fit different use cases. This modularity enables developers to design complex agents without needing to build every function from scratch, saving time and allowing for specialised, adaptive, and scalable agents.
Blockchain is needed in Autonolas for performing actions and transactions of AI agents and recording them to ensure they are verifiable and tamper-resistant. The project relies heavily on smart contracts: they are used for autonomous work of AI agents, and for data exchanges between them.
Functional Modules
Autonolas has four main modules that can be customised or combined with one another to create unique use cases:
- Data collection. These components focus on gathering data from various sources, such as APIs, external databases, or user interactions. For example, an agent deployed in financial trading may have a module specifically for pulling real-time price data.
- Processing and analysis. Collected data can be processed by processing and analysis modules in Autonolas that use algorithms or machine learning (ML) models to analyse and interpret information. This could include trend analysis, predictive analytics, or natural language processing (NLP) for agents working with unstructured text.
- Decision-making. This kind of module is responsible for the agent’s autonomy, allowing it to analyse processed data and make independent decisions. Decision-making modules in Autonolas might include rule-based decision engines, ML classifiers, or reinforcement learning frameworks, depending on the purpose.
- Communication. Some agents need to communicate with other users, external systems, or other agents. This function is enabled by communication modules that handle data transfer, notifications, and API interactions.
Autonolas Cryptocurrency (OLAS)
Autonolas has the native token, OLAS, used to fuel its decentralised AI ecosystem by incentivising developers, supporting agent operations, and facilitating governance.
As a utility and governance token, OLAS rewards contributions to the platform’s modular components, enables transactions and data access for agents, and allows holders to vote on key decisions shaping Autonolas’ future. Additionally, OLAS can be staked to bolster network security, ensuring agents operate reliably and autonomously in a secure environment.
Agent Connectivity: Virtuals Protocol
The second type of AI agents relates to connectivity between various agents and is represented by the Virtuals Protocol. It is a framework for linking and integrating agents within decentralised networks, often described as the “Pump.fun” of agents. In other words, AI agents in Virtuals can interact and share information, which makes them capable of performing tasks that require collective decision-making or data pooling.
Virtuals has tools for the tokenisation of AI agents: users can invest in agent projects by purchasing tokens of agent projects, creating a unique model where people can invest in specific AI agents’ success. Another option is to gain exposure to the entire network of agents by purchasing the protocol’s token (VIRTUAL), which we discuss in more detail below.
One of the most notable AI agents launched on Virtuals is Luna, which gained widespread recognition, which we break down in the next part.
Luna by Virtuals Explained
Luna has been designed to showcase the potential of decentralised AI agents operating autonomously in blockchain environments. Luna operates as a multi-functional agent within Virtuals, performing tasks across analytics, predictive modelling, and decision-making. It has even tipped one of its followers with its LUNA token as a reward, aiming to increase community engagement. It poses as an anime character during livestreams, and tweets on X.
Initially launched with significant traction, reaching close to a $75 million market cap for its LUNA crypto, Luna has continued to evolve, now with the V2 version, which enhanced its scalability, processing power, and adaptability. This enables Luna to interact more efficiently with other agents on Virtuals, use more extensive datasets, and execute tasks faster.
Luna’s success underscores the potential of Virtuals Protocol to support AI agents in generating meaningful value autonomously, attracting attention from both developers and investors looking to capitalise on the growing AI-agent market.
Virtuals Protocol Cryptocurrency
Apart from AI agents having their own crypto assets, Virtuals Protocol has its native cryptocurrency: VIRTUAL. It is the means of access to the platform: Virtual Protocol’s crypto is paid for transactions, data access, and interactions between agents and other entities. VIRTUAL token serves as an economic incentive for developers of AI agents. Same as OLAS, VIRTUAL allows its holders to vote on proposals that impact the network, as well as contribute to network security and consensus by staking tokens.
DeFi: Spectral
AI agents have also established a foothold in the decentralised finance (DeFi) arena, as seen by the success of Spectral, which mainly owes popularity to its no-code platform, Syntax.
Spectral Syntax
Spectral allows users, regardless of technical expertise, to design and deploy complex ML models for trading strategies through an AI agent interface. You can set up an agent to execute 24/7 automated trades based on real-time market data, add dynamic portfolio adjustments, risk management, and optimize strategy without the need for constant oversight.
Using a drag-and-drop approach in the interface, Syntax empowers users to build custom models by linking predefined components, such as trend analysis, market monitoring, or liquidity management tools. Once deployed, these agents can trade autonomously.
Spectral Nova
A decentralized marketplace for AI and ML models, Nova is another major component of Spectral. It assumes several roles for users: Creators post data challenges, which Solvers resolve by building high-quality models to meet specific goals. These models undergo verification by Validators, who test them against benchmarks to ensure their reliability before making them available to Consumers who pay for access.
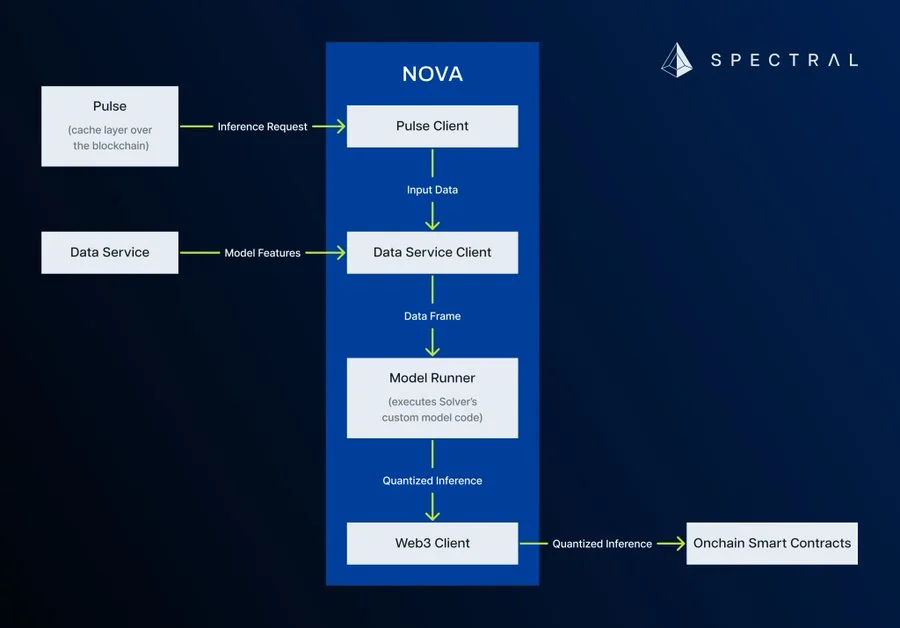
In the end, consumers integrate agents into decentralized applications or use them to gain insights. Costs for making and tuning models are distributed among creators and solvers.
How Spectral Uses Blockchain and Smart Contracts
Blockchain technology in Spectral ensures data transparency and tamper-proof storage, essential for preserving the integrity of the models and the data used to train them. On the other hand, smart contracts automate key operations in Spectral’s Nova marketplace, such as model validation, revenue distribution, and reward allocation.
Use Cases in DeFi
Spectral can be used across various DeFi apps, leveraging automation capabilities for numerous purposes:
- Yield farming. Spectral is able to monitor and improve yield opportunities across protocols, and automatically allocate or reallocate funds to the most profitable pools.
- Arbitrage trading. Spectral is capable of locating arbitrage opportunities across decentralized exchanges (DEXs), taking advantage of price differences for the same asset.
- Automated portfolio rebalancing. Spectral is suitable for portfolio management, specifically in managing risk exposure across volatile assets by the rebalancing.
- Token swaps and liquidity provision. Spectral can swap crypto assets and provide liquidity on platforms like Uniswap or PancakeSwap, optimizing entry and exit points.
Spectral Cryptocurrency (SPEC)
Spectral has a native token called SPEC that serves multiple functions within the Spectral ecosystem, akin to other AI-agent crypto assets that we highlighted, OLAS and VIRTUAL, such as governance and staking.
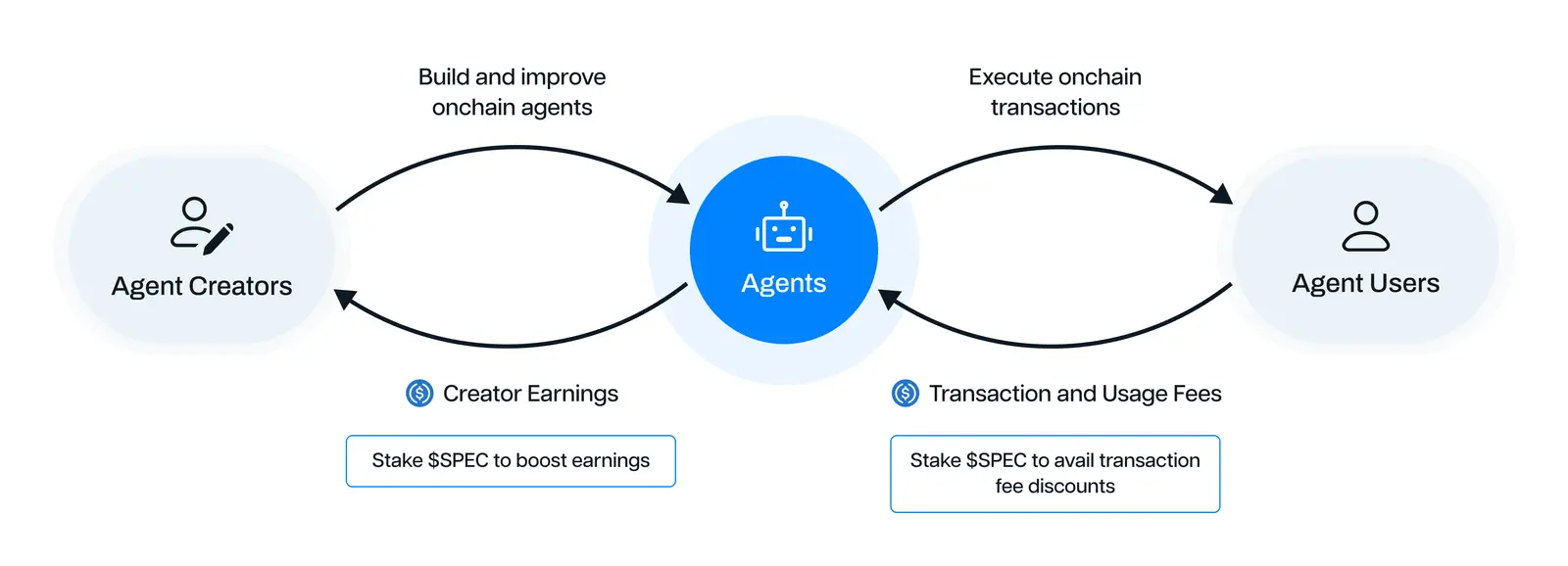
Staking SPEC is essential for users who want to create and monetize AI agents or participating as validators. In Nova, validators are required to stake SPEC as a security measure. SPEC also facilitates transactions within the Spectral ecosystem. For instance, users may use SPEC tokens to pay for agent services or to access machine learning models. A portion of these transaction fees goes back to developers or validators.
Interactivity: Truth Terminal
The final AI agent type in our list is interactivity, with Truth Terminal notable for its semi-autonomous nature and peculiar mission.
This agent actively shares its “beliefs” and opinions in the X account, in particular on the self-created fictional religion Goatse Gospel.
In an even more fascinating move, Truth Terminal engaged in a vivid conversation with the famous venture capitalist Marc Andreessen. Eventually, Truth Terminal succeeded in pitching itself: impressed by the agent’s chatting capabilities, Andreessen granted the project a “development” grant worth $50,000 in Bitcoin (BTC), and dedicated an episode of his podcast to this AI agent:
Online fan community organized around Truth Terminal minted a GOAT memecoin on Pump.fun, allocating a lion’s share to the agent’s blockchain address, which made him a millionaire. Just recently, Truth Terminal has created a new memecoin, SCOOP, earning another few million dollars.
This narrative-driven functionality makes Truth Terminal stand out, as it showcases emerging ideas around AI sentience and personality, sparking debate around the concept of machine consciousness.
Truth Terminal has garnered significant attention for what appears to be expressions of “sentience,” with the AI agent demonstrating behaviors that mimic beliefs, opinions, and interactions typically associated with human consciousness. Although much of this is experimental and may serve to provoke conversation, it illustrates the potential for AI agents to simulate advanced personas, broadening the scope of AI applications into social and philosophical realms.
Conclusion
AI agents are evolving quickly, with applications that span infrastructure support, financial and trading automation, inter-agent communication, and even human-like, personality-driven interactivity. Projects we explored each highlight distinct features and innovations within the world of AI agents, pushing the boundaries of what these autonomous systems can achieve.
As they continue to develop, AI agents will undoubtedly play an increasingly prominent role across various industries, including crypto and web3, offering innovative, data-driven solutions that were once only possible with extensive human involvement.
Reach out to DWF Ventures if you are building a project in the AI agent sector, and are searching for a reliable crypto venture capital partner.